হাইপারস্পেকট্রাল ইমেজ শ্রেণিবিন্যাসের জন্য হায়ারার্কিকাল কনভোলিউশনাল বৈশিষ্ট্যগুলি অন্বেষণ
নভেম্বর 2018 এর জন্য টিজিআরএস নিবন্ধ।
এই কাগজের মূল কাজটি একটি বৈশিষ্ট্য শিখতে হয়, যা বর্ণালী বৈশিষ্ট্য এবং গভীর জালের স্থানিক বৈশিষ্ট্যগুলির সাথে একত্রিত করে বর্ণালী-স্থানগত বৈশিষ্ট্য উপস্থাপনা অর্জন করতে পারে। এই উপায়ে প্রাপ্ত বৈশিষ্ট্যের স্থানটিতে একই ধরণের দূরত্ব খুব কাছাকাছি হলেও একই ধরণের দূরত্ব খুব বেশি নয় class শ্রেণিবিন্যাসের জন্য এসভিএমকে খাওয়ানোর জন্য এই বৈশিষ্ট্যটি ব্যবহার করুন, এবং প্রভাবটি এসওটিএ পৌঁছাতে পারে।
ভূমিকা:
হাইপারস্পেকট্রাল রিমোট সেন্সিং চিত্রগুলির শ্রেণিবিন্যাস (এইচএসআই, হাইপারস্পেকট্রাল ইমেজ) একটি গুরুত্বপূর্ণ বিষয়। প্রাথমিক পদ্ধতিটি হ'ল প্রতিটি পয়েন্টের সমস্ত চ্যানেল, অর্থাৎ এর ফ্রিকোয়েন্সি বর্ণালী, ইনপুট বৈশিষ্ট্য হিসাবে এবং তারপরে শ্রেণিবদ্ধকরণের জন্য এসভিএমের মতো পদ্ধতিগুলি ব্যবহার করা। অবশ্যই, প্রভাবটি অসন্তুষ্টিজনক, কারণ বাস্তবে একই ধরণের বিভিন্ন বর্ণালী এবং একই বর্ণালী রয়েছে reality অবস্থা। পরে, লোকে বর্ণালী-স্থানিক বৈশিষ্ট্যগুলি বের করতে স্থানিক বৈশিষ্ট্যগুলি ব্যবহার করে, যা ব্যাপকভাবে উন্নত করা হয়েছে। এছাড়াও, রূপচর্চা ব্যবহার করে টেক্সচার বৈশিষ্ট্যগুলি বের করার জন্য বিভিন্ন পদ্ধতি রয়েছে।
সাম্প্রতিক বছরগুলিতে, গভীর শিক্ষার অ্যালগরিদমগুলির উত্থান মানুষকে গভীর নেটওয়ার্ক পদ্ধতিতে পরিচালিত করেছে, যা মূলত স্থানীয় প্যাচ-ভিত্তিক নমুনাগুলির উপর ভিত্তি করে এই পয়েন্টের বৈশিষ্ট্যগুলি সন্ধান করতে এবং এইচএসআই স্পেসের পয়েন্টগুলিকে পরবর্তী শ্রেণিবিন্যাসের জন্য স্থান বৈশিষ্ট্যে রূপান্তরিত করে। এই ফলাফলটি বর্ণালী এবং স্থানের দ্বৈত বৈশিষ্ট্য নিয়ে আসে।
লেখক বিশ্বাস করেন যে সিএনএন-ভিত্তিক এইচএসআই শ্রেণিবদ্ধকরণ অ্যালগরিদমের দুটি সমস্যার সমাধান করতে হবে। একটি হ'ল নমুনাটি ছোট এবং নেটওয়ার্ক ছোট, সুতরাং সাধারণকরণের ক্ষমতা কম poor অন্যটি হ'ল কেবলমাত্র শেষ স্তরটি বৈশিষ্ট্যগুলি নিষ্কাশন করতে, উচ্চ-স্তরের তথ্য বজায় রাখতে ব্যবহৃত হয়, তবে সূক্ষ্ম ধরণের বিবরণ হারিয়ে যায়। এই নিবন্ধটি এই দুটি সমস্যা সমাধানের উদ্দেশ্যে করা হয়েছে।
পদ্ধতি
বৈশিষ্ট্য নিষ্কাশনের
এই পদক্ষেপটি গভীর স্থানিক বৈশিষ্ট্যগুলি নিষ্কাশন করা যা শেল্ফ সিএনএন ব্যবহার করে প্রয়োগ করা হয়। আপনি এই সময়ে যা পান তা স্থানিক বৈশিষ্ট্য।
মেট্রিক লার্নিং-ভিত্তিক বৈশিষ্ট্য ফিউশন, বৈশিষ্ট্য শিখন এবং শ্রেণিবদ্ধ প্রশিক্ষণ
This এই পদক্ষেপটি স্থানিক এবং বর্ণালী বৈশিষ্ট্যগুলি ফিউজ করা এবং তারপরে বিকল্প বৈশিষ্ট্য শিখন এবং শ্রেণিবদ্ধ প্রশিক্ষণ। এই প্রক্রিয়াটি মেট্রিক শিক্ষার কাঠামোর ব্যবহার করে প্রয়োগ করা হয়।
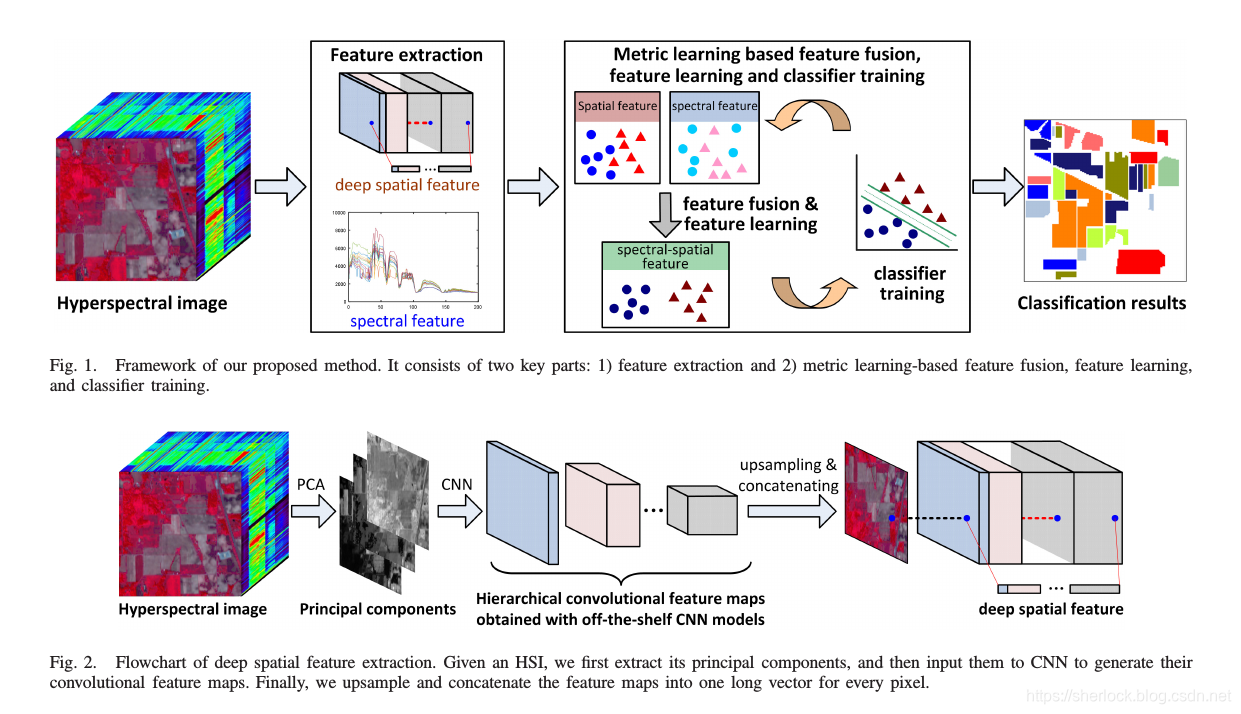
এখানে সিএনএন সহ বৈশিষ্ট্য নিষ্কাশন সাধারণ পদ্ধতির মতো নয়, শ্রেণিবদ্ধকরণ নেটওয়ার্কের শেষ দুটি এফসি স্তরগুলির ভেক্টরগুলিকে বৈশিষ্ট্য হিসাবে ব্যবহার করে, কারণ লেখক বিশ্বাস করেন যে এই ভেক্টরটিতে কেবলমাত্র উচ্চ-অর্ডার সিমেটিক বৈশিষ্ট্য এবং কম স্থানিক বৈশিষ্ট্য রয়েছে। এখানে দৃষ্টিভঙ্গি সমস্ত বৈশিষ্ট্য মানচিত্রকে একই আকারে উপস্থাপন করা এবং তারপরে সেগুলিকে একত্রিত করা হয় a চূড়ান্ত ফলস্বরূপ, এই বহু-চ্যানেল গ্রাফের প্রতিটি পয়েন্টের সমস্ত চ্যানেলগুলি আমাদের প্রয়োজনীয় স্থানিক বৈশিষ্ট্য।
পরবর্তী পদক্ষেপটি একটি মেট্রিক শেখার প্রক্রিয়া The মেট্রিক ক্ষয় ফাংশনটি ক্লাসের মধ্যে অন্তর এবং ক্লাসের মধ্যে অন্তরের একটি ফাংশন, যা RELIEF অ্যালগরিদমের মতো। শ্রেণিবদ্ধকারী এসভিএম ব্যবহার করে এবং চূড়ান্ত ফলাফলটি অপ্টিমাইজেশনের মাধ্যমে প্রাপ্ত হয়।
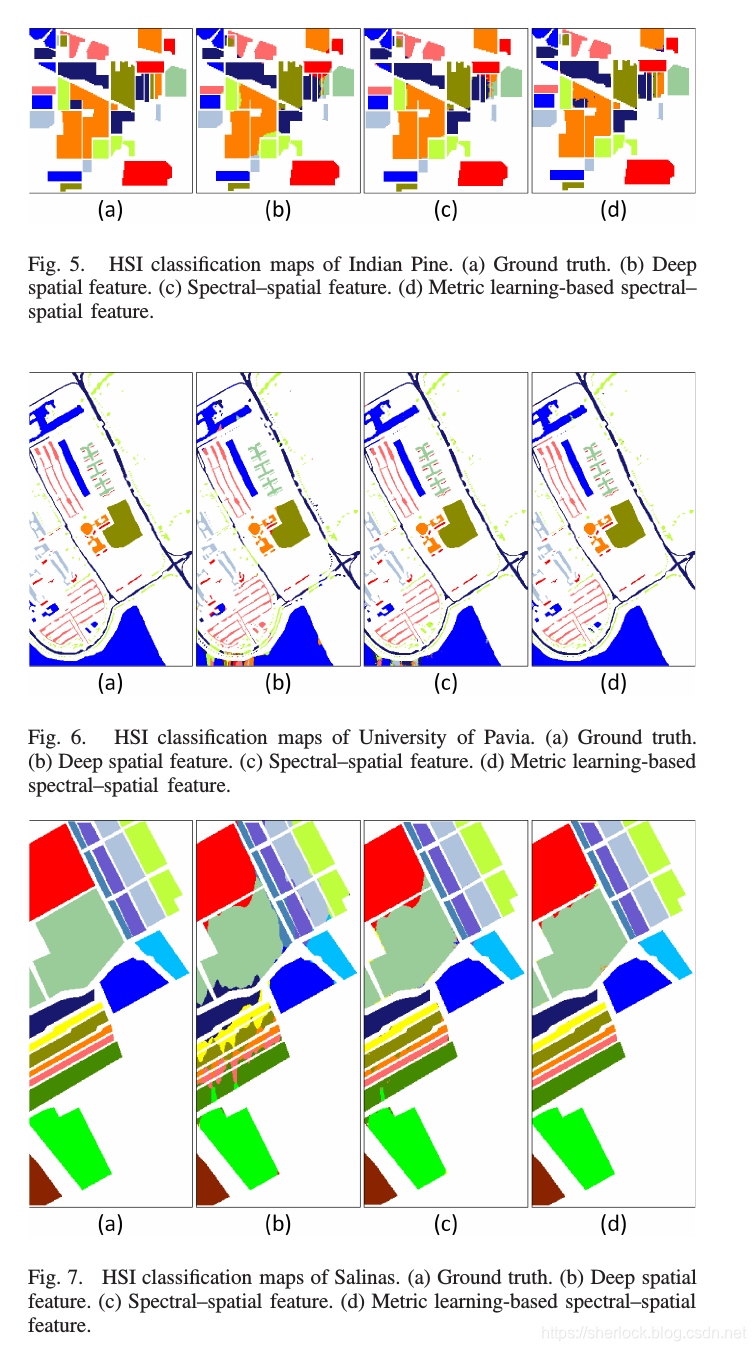
পরীক্ষামূলক ফলাফলগুলি উপরের চিত্রটিতে দেখানো হয়েছে এবং তারা পাভিয়া বিশ্ববিদ্যালয় এবং ভারতীয় পাইনের মতো পাবলিক ডেটাসেটগুলিতে সোটা স্তরে পৌঁছেছে।
এটি উল্লেখ করার মতো যে এই পদ্ধতির গতিটি আরও ভাল, কারণ কোনও প্রশিক্ষণ প্রক্রিয়া নেই, সিএনএন প্রি-ট্রেন অফ-শেল্ফ ব্যবহার করে, তাই এটি আরও দ্রুত।
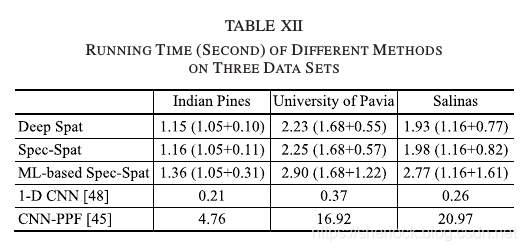
Exploring Hierarchical Convolutional Features for Hyperspectral Image Classification
TGRS article for November 2018.
The basic task of this paper is to learn a feature, which can be combined with the spectral features and the spatial features of the deep net to obtain a spectral-spatial feature representation. In the feature space obtained in this way, the distance of the same kind is closer, but the distance of the same kind is not far. Using this feature to feed the SVM for classification, the effect can reach SOTA.
Introduction: The
classification of hyperspectral remote sensing images (HSI, HyperSpectral Image) is an important issue. The initial method is to use all channels of each point, that is, its frequency spectrum, as input features, and then use methods such as SVM to classify. Of course, the effect is unsatisfactory, because in reality there are different spectra of the same type and the same spectrum Case. Later, people used spatial features to extract spectral-spatial features, which has been greatly improved. In addition, there are methods to extract texture features using morphology.
In recent years, the emergence of deep learning algorithms has led people to turn to deep network methods, which are mainly based on local patch-based samples to extract features of this point, and convert points in HSI space to feature space for later classification. This result brings the dual characteristics of spectrum and space.
The author believes that CNN-based HSI classification algorithm has two problems to be solved. One is that the sample is small and the network is small, so the generalization ability is poor; the other is that only the last layer is used to extract features, retaining high-level information, but losing fine-grained details. This article is intended to address these two issues.
Method
This step of feature extraction is to extract deep spatial features, which is implemented using of-the-shelf CNN. What you get at this time is the spatial feature.
metric learning-based feature fusion, feature learning, and classifier training.
This step is to fuse spatial and spectral features, and then alternate feature learning and classifier training. This process is implemented using the framework of metric learning.
The feature extraction with CNN here is not like the ordinary method, using the vectors of the last two FC layers of a classification network as features, because the author believes that this vector has only high-order semantic features and less spatial features. The approach here is to upsample all feature maps to the same size and then concat them. As a final result, all channels at each point of this multi-channel graph are the spatial features we need.
The next step is a metric learning process. The metric loss function is a function of the interval between classes and the interval between classes, similar to the RELIEF algorithm. The classifier uses SVM, and the final result is obtained through optimization.
The experimental results are shown in the figure above, and they have reached the SOTA level on public datasets such as PAVIA UNIVERSITY and Indian pines.
It is worth mentioning that the speed of this method is also better, because there is no training process, CNN uses pretrain off-the-shelf, so it is faster.
0 comments:
Post a Comment