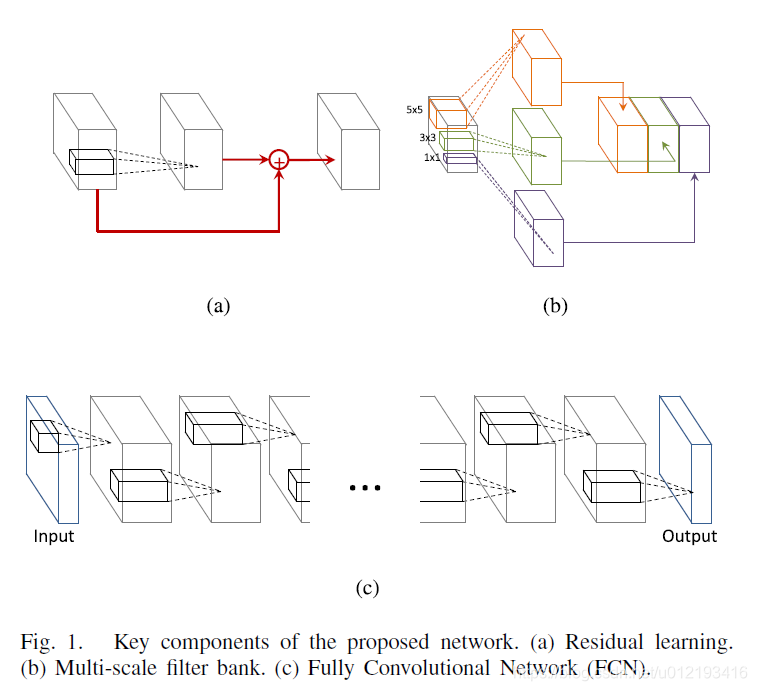
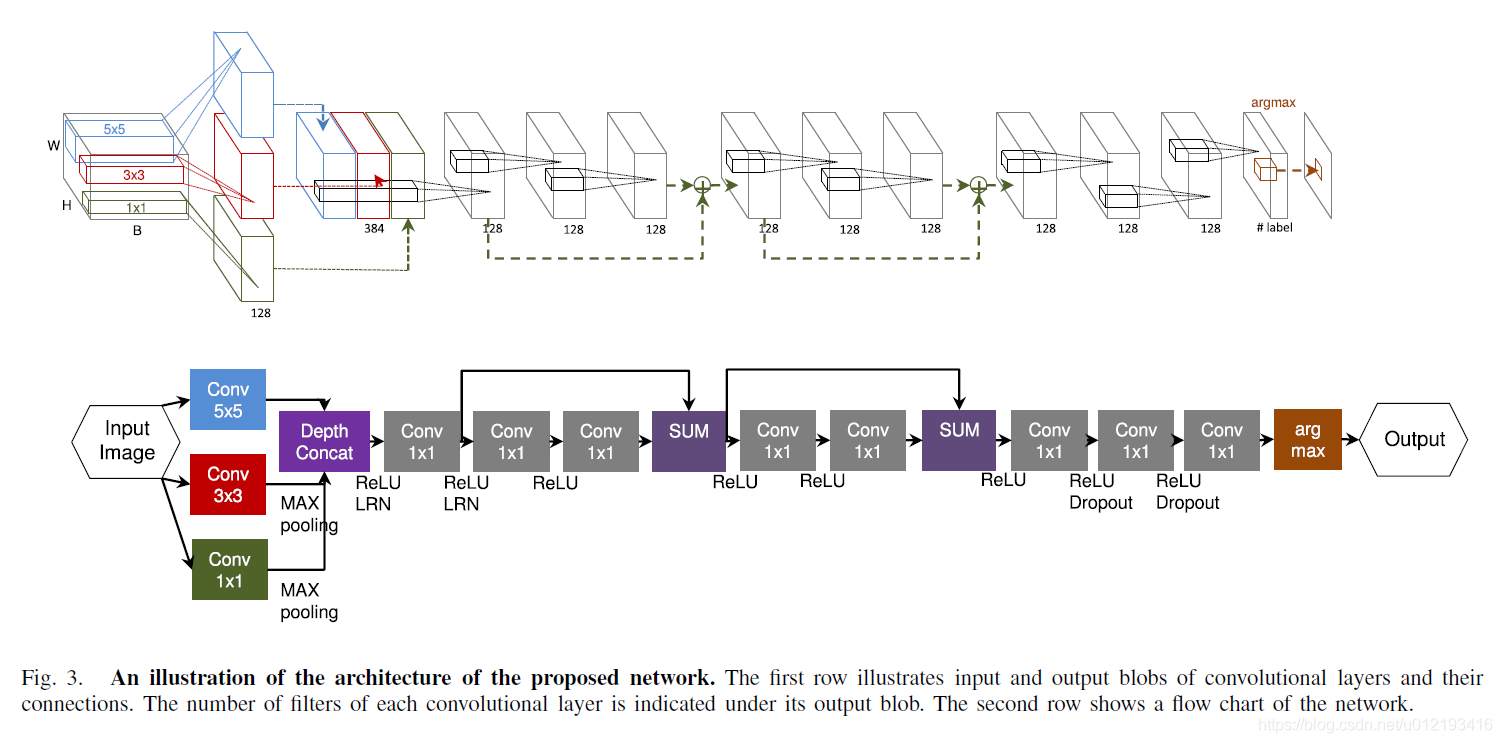
হাইপারস্পেকট্রাল চিত্রগুলির বর্ণালী মাত্রার হ্রাস সাধারণত প্রাথমিকভাবে মূল উপাদান বিশ্লেষণ (পিসিএ) [9] এর মতো কৌশলগুলি ব্যবহার করে ক্ষুদ্র নেটওয়ার্কগুলিতে ইনপুট ডেটা ফিট করার জন্য সম্পাদিত হয় balanced স্থানীয় বৈষম্যমূলক এম্বেডিং (বিএলডিই) [৩], যুগলবন্দি সীমাবদ্ধতা বৈষম্যমূলক বিশ্লেষণ এবং ননজিগেটেভ স্পার্স ডাইভারজেন (পিসিডিএ-এনএসডি) [১০], ইত্যাদি
এই পিসিএ-জাতীয় মাত্রা হ্রাস অপারেশন একটি প্রাক প্রক্রিয়াজাতকরণ পদ্ধতি যা সাধারণত পূর্বের পদ্ধতিগুলিতে ব্যবহৃত হয়। প্রকৃতপক্ষে, এমনকি সিএনএন পদ্ধতিটি শ্রেণিবদ্ধ প্রশিক্ষণ দেওয়ার জন্য বর্ণালী বৈশিষ্ট্যগুলির সাথে মার্জ করার আগে স্থানিক বৈশিষ্ট্যগুলি আহরণের জন্য ব্যবহৃত হয়েছিল। অতএব, লেখক মূলত এই পয়েন্টটি উল্লেখ করেছেন। লেখক বিশ্বাস করেন যে তাদের সিএনএন সরাসরি এই দুটি বৈশিষ্ট্যই বের করতে পারে। এটিও এই নিবন্ধটির অবদান।
মাল্টি-স্কেল ফিল্টার ব্যাংকটি মূলত স্থানীয় স্থানীয় কাঠামোগত পাশাপাশি স্থানীয় বর্ণালী পারস্পরিক সম্পর্ককে কাজে লাগাতে ব্যবহৃত হয় fact বাস্তবে, এটি আবিষ্কারের সরল সংস্করণ। । ।
পরীক্ষামূলক পরীক্ষাটি তিনটি ওপেন সোর্স ডেটাसेट ব্যবহার করে:
ইন্ডিয়ান পাইাইনস ডেটাসেটের জন্য 145 × 145 পিক্সেল
, পাভিয়া ডেটাসেট বিশ্ববিদ্যালয়ের জন্য 610 × 340 পিক্সেল,
স্যালিনাস ডেটাসেটের জন্য 512 × 217
ভারতীয় পাইনেস, পাভিয়া ইউ, স্যালিনাস।
এই নিবন্ধটি গভীর নেটওয়ার্কের সাথে এই কাজটি করার জন্য প্রথম নিবন্ধ বলে দাবি করেছে এবং এটি একই সাথে স্থানিক বর্ণালী বৈশিষ্ট্যগুলি বের করতে পারে এবং প্রতিষ্ঠা এবং রেসনেটের মতো অনেকগুলি কাঠামো ব্যবহার করতে পারে।
নেটওয়ার্ক মডেলটি নিম্নরূপ:
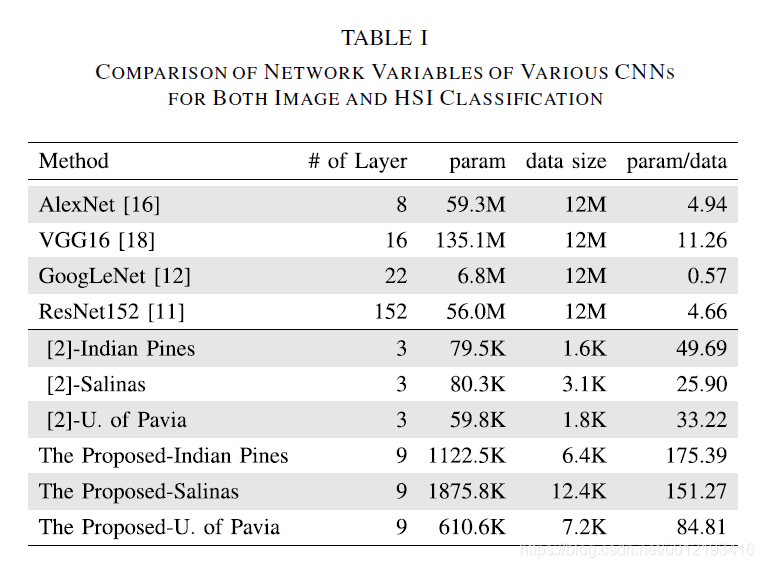
প্রশিক্ষণ পদ্ধতিটি নিম্নরূপ:
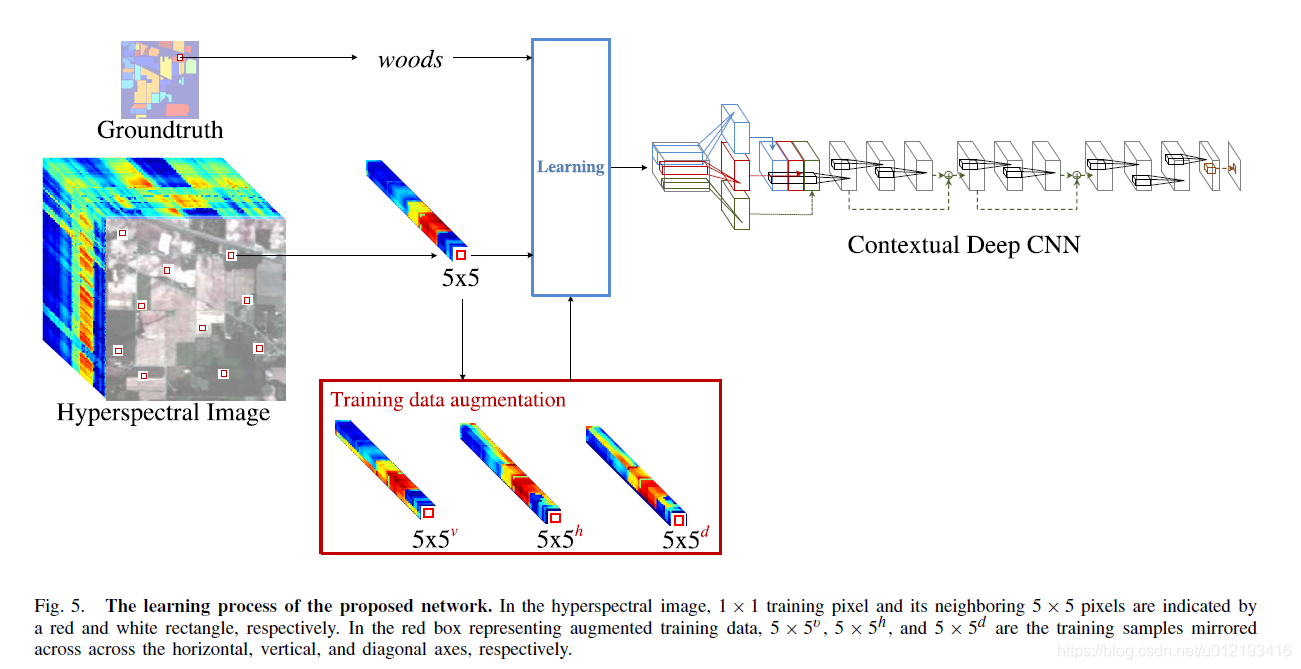
প্রশিক্ষণ সেট এবং পরীক্ষার সেট পেতে হাইপারস্পেক্ট্রামে এলোমেলোভাবে পিক্সেল নমুনা সংগ্রহ করা পয়েন্ট এবং তাদের 5x5 পাড়ার প্যাচগুলি ইনপুট হিসাবে ব্যবহৃত হয়, এবং সংশ্লিষ্ট গ্রাউন্ড-সত্য প্রশিক্ষণের জন্য উপরের নেটওয়ার্কে ইনপুট হওয়ার জন্য একটি রেফারেন্স হিসাবে ব্যবহৃত হয়। পরীক্ষার ফলাফলগুলিও এই জাতীয় বহু-চ্যানেল ছোট প্যাচগুলির সাথে সম্পন্ন হয়।
অতিরিক্ত চাপ এড়াতে লেখক প্যাচটি অনুভূমিকভাবে, উল্লম্বভাবে এবং তির্যকভাবে আয়রনের হারের চারগুণ অর্জন করতে মিরর করেছেন।
পরীক্ষামূলক ফলাফল:
নেটওয়ার্ক-ভিত্তিক পদ্ধতির সাথে তুলনা করে, এই নিবন্ধের ফলাফলগুলি এসওটিএ পৌঁছেছে।
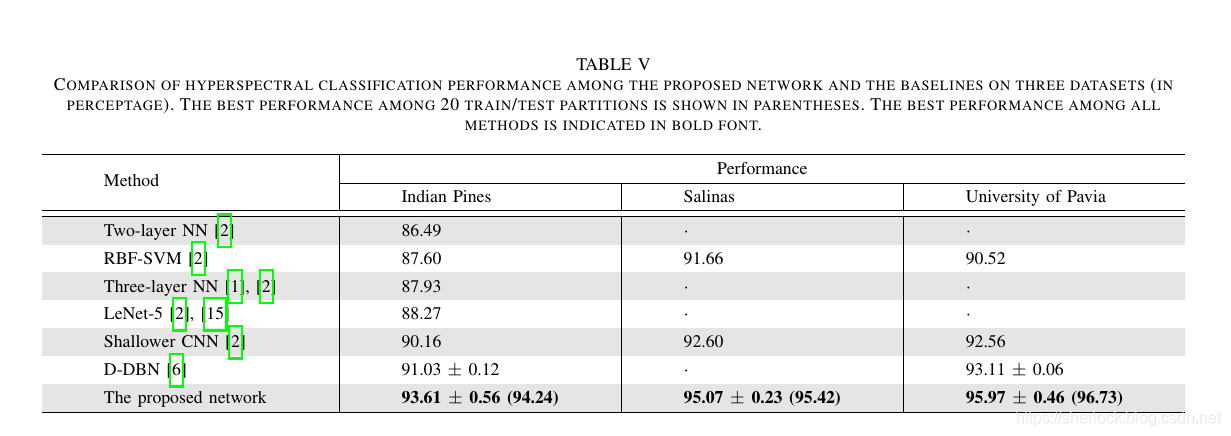
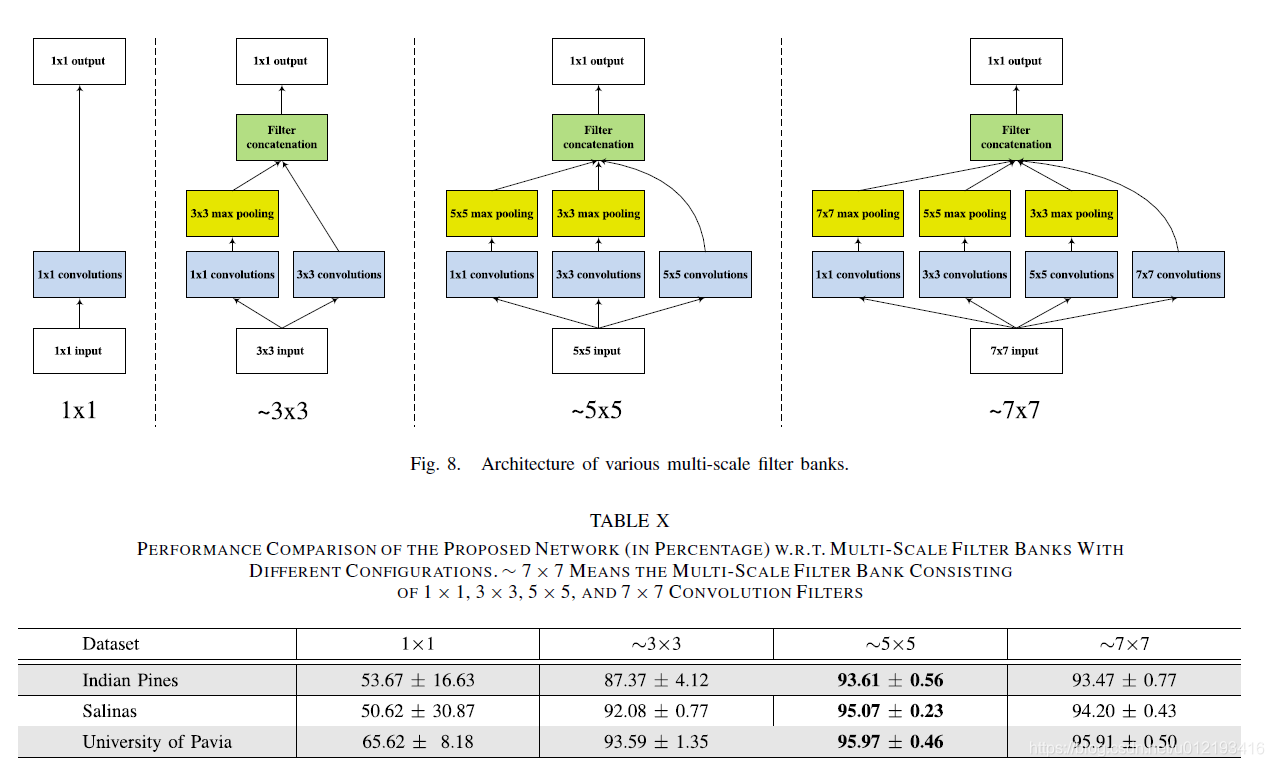
Going Deeper with Contextual CNN for Hyperspectral Image Classification
2017 TIP article.
This article mainly proposes a new network model, which is deeper and adds the method of Googlenet's multiple scales (kernel = 1,3,5) in the same layer to achieve deeper and wider. The main problem here is that the existing CNN method has a smaller and simpler network structure in the HSI classification problem and cannot achieve the optimal result. The author of this article uses a deeper and wider network (in fact, an inception structural unit is added) to implement HSI classification. One of its benefits is that it can learn the characteristics of spatial and spectral at the same time. In this deep network, the structure of FCN, ResNet, and multi-scale filter, which is the structure of inception, are used. As shown: Thus
, the reduction of the spectral dimension of the hyperspectral images is in general initially performed to fit the input data into the small-scale networks by using techniques, such as principal component analysis (PCA) [9], balanced local discriminant embedding (BLDE) [3], pairwise constraint discriminant analysis and nonnegative sparse divergence (PCDA-NSD) [10], etc.
This PCA-like dimension reduction operation is a pre-processing method commonly used in previous methods. In fact, even the CNN method was used to extract spatial features before merging with spectral features to train the classifier. Therefore, the author mainly mentioned this point. The author believes that their CNN can directly extract both of these features. This is also a contribution of this article.
The multi-scale filter bank is basically used to exploit various local spatial structures as well as local spectral correlations. In fact, it is a simplified version of inception. . .
The test experiment uses the three open source datasets:
145 × 145 pixels for the Indian Pines dataset,
610 × 340 pixels for the University of Pavia dataset,
512 × 217 for the Salinas dataset
Indian pines, pavia U, Salinas.
This article claims to be the first article to do this task with a deeper network, and can simultaneously extract spatial-spectral features, and use many structures such as inception and ResNet.
The network model is as follows:
The training method is as follows:
Randomly sample the pixels in the hyperspectrum to obtain a training set and a test set. The collected points and their 5x5 neighborhood patches are used as input, and the corresponding ground-truth is used as a reference to be input into the above network for training. The test results are also done with such multi-channel small patches.
In addition, in order to avoid overfitting, the author mirrored the patch horizontally, vertically, and diagonally to achieve 4 times the rate of augmentation.
Experimental results:
Compared with the network-based method, the results of this article reach SOTA.
0 comments:
Post a Comment