Foreword
This document summarizes the basic knowledge of concept definition, analysis and classification of hyperspectral remote sensing images. The first part introduces the general principle and knowledge of hyperspectral image, the second part introduces the noise problem of hyperspectral image; the third part introduces the data redundancy problem of hyperspectral image and the method of data dimension reduction to solve the redundancy; The problem of mixed pixels in hyperspectral images introduces a certain degree of spectral demixing. The fifth and sixth sections introduce the characteristics, processes, and algorithms of supervised classification and sub-supervised classification of hyperspectral images, respectively.
1. Basic introduction
Hyperspectral remote sensing is a multi-dimensional information acquisition technology that combines imaging technology and spectral technology, and simultaneously detects the two-dimensional collection space of the target and one-dimensional spectral information to obtain continuous, narrow-band image data with high spectral resolution.
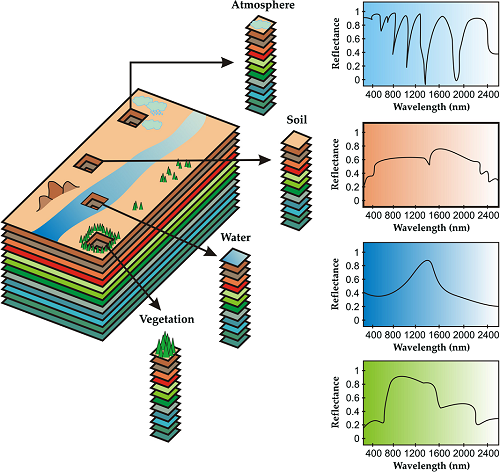
Hyperspectral image and a high resolution image , multi-spectral images different.
Hyperspectral recognition advantages:
- The spectral resolution is high and the number of bands is high. It can obtain almost continuous spectral characteristic curves of ground features, and can select or extract specific bands to highlight target features according to needs;
- At the same spatial resolution, the spectral coverage is wider, and the response characteristics of ground objects to electromagnetic waves can be detected;
- Multiple bands, which facilitates the mutual correction between the bands;
- Quantitative continuous spectral curve data provides the conditions for the introduction of image classification by the model of ground surface spectral mechanism;
- Contains a wealth of radiation, space and spectral information, is a comprehensive carrier of a variety of information.
Difficulties in identifying hyperspectral:
- Large amount of data, the image contains dozens to hundreds of bands, the amount of data is hundreds of times that of the single-band remote sensing image; there is a lot of redundancy in the data, and improper processing will affect the classification accuracy;
- The classification of hyperspectral images requires higher accuracy of spectral calibration and reflectance conversion on the one hand, and it is difficult to preprocess image data due to the complex imaging mechanism and huge data volume, including atmospheric correction, geometric correction, Spectral calibration and reflectance conversion;
- There are many bands and high correlation between bands, so the number of training samples required for classification has greatly increased, and the training parameters obtained are often unreliable due to insufficient training samples (dimensional disaster);
- Conventional remote sensing processing models and methods cannot meet the needs of hyperspectral image classification. One of the main problems is the parameter estimation problem of the statistical classification model, which requires high selection of spectral features.
Hughes phenomenon in hyperspectral image classification:
Hughes phenomenon: the limited data sample in the machine learning problem, it is necessary in the high-dimensional feature space (each feature can take on a range of possible values) in learning a "state of nature" (possibly infinite distribution), requires considerable The amount of training data contains some sample combinations. Given a fixed number of training samples, its predictive power decreases with increasing dimensions.
In hyperspectral remote sensing images, when the number of training samples is limited, the classification accuracy increases first with the number of image bands. After reaching a certain extreme value, the classification accuracy decreases with the number of bands.
The traditional remote sensing image analysis uses image spatial information. The core of hyperspectral image analysis is spectral analysis . Hyperspectral remote sensing data is a spectral image cube. Its main feature is the integration of image space dimension and spectral dimension information. Compared with a single band, it has one-dimensional spectral information. While acquiring the surface space image, the spectral information of each object corresponding to each pixel will be obtained.
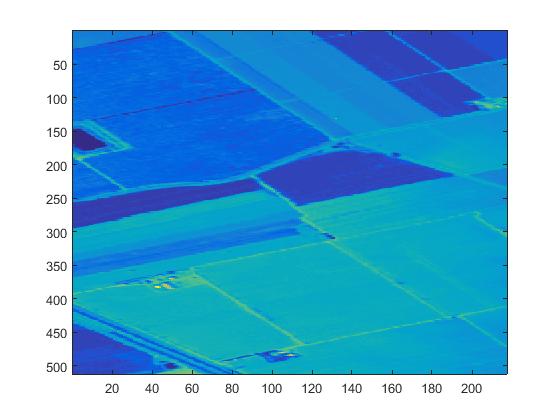
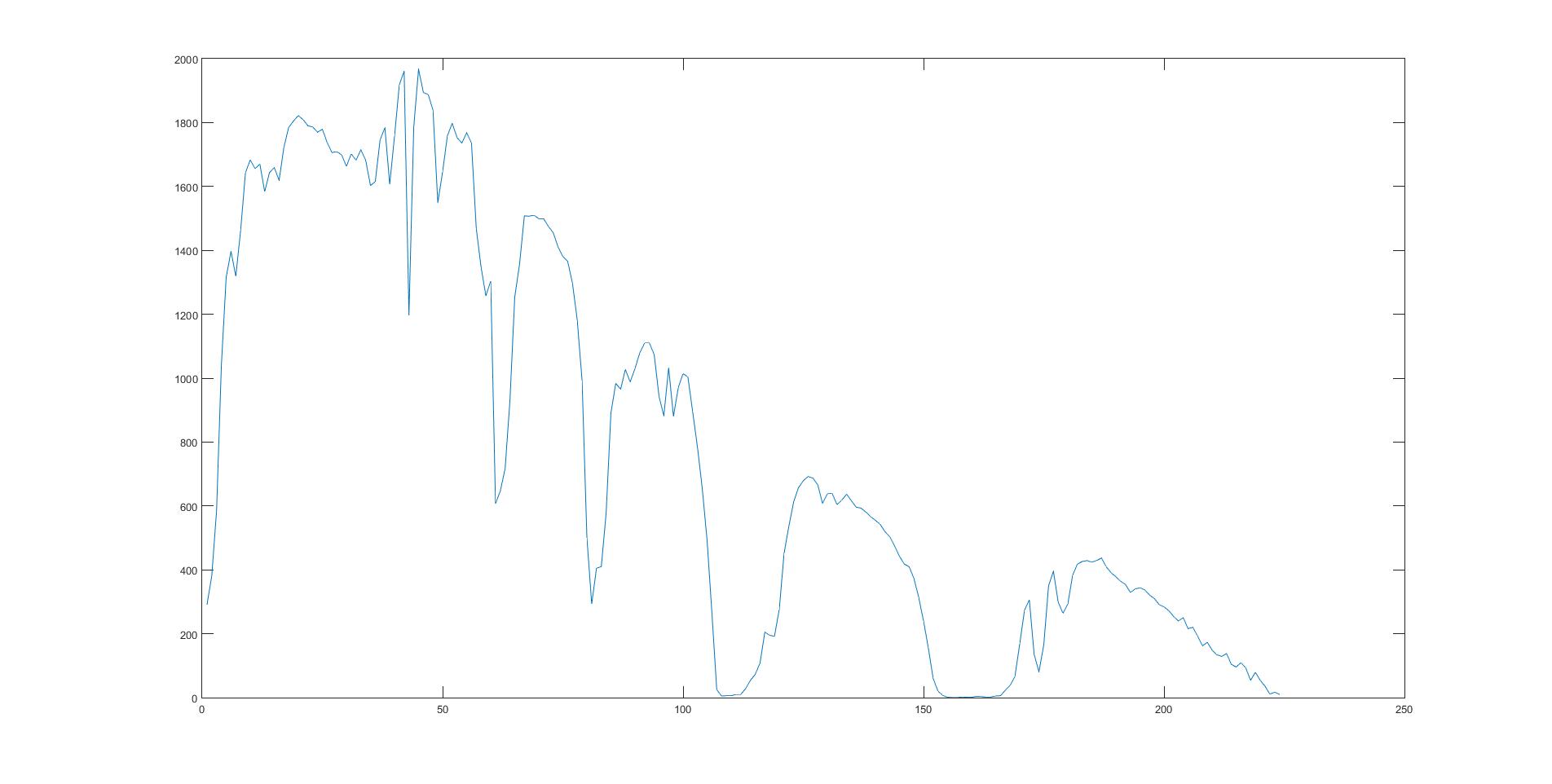
The figure above is the remote sensing image of a certain band and the spectral characteristic of a pixel.
2. Noise processing of hyperspectral data
Fine spectral data of hyperspectral images can fully reflect the subtle features of the ground feature spectrum. According to the relative differences in the spectral characteristics of different features, the feature classification can be realized, and target detection and fine classification can be realized.
However, due to the lack of light imaging energy due to the dense channel of the imaging spectrometer, it is more difficult to improve the signal-to-noise ratio (SNR) of hyperspectral images compared to panchromatic images . In the process of acquiring image data, the features of the ground features are prone to "distortion" under the influence of noise. In addition, due to the large amount of hyperspectral data, in the fine classification process, it is often necessary to perform dimensionality reduction processing, and in the process of dimensionality reduction, it is necessary to retain the signal and compression noise to the greatest extent, so accurate noise evaluation is necessary. In addition, noise has the most direct impact on the results of fine classification. Therefore, noise assessment is needed for hyperspectral data.
The main noise is considered to be Gaussian white noise, which is divided into additive noise and multiplicative noise.
There are three main methods for noise evaluation: laboratory method, dark current method and image method. Because the first two methods are difficult to implement in experiments, the third one, the image method , is widely used .
The image method is divided into the following types:
- Uniform area method (HA)
The main idea is to select more than four uniform regions from the image and obtain the estimated value of image noise by calculating the average of the standard deviation of these uniform regions.
Disadvantages: Uniform region selection needs to be performed manually and cannot be automated; uniform regions that meet the conditions do not exist in most remote sensing maps; noise estimation of subregions cannot represent the noise of the entire image.
- Geostatistical Method (GS)
The main idea: select several uniform narrow bands from the image, and realize the estimation of image noise by calculating the semi-variance function of these narrow bands. To a certain extent, the spatial correlation of imaging spectral data is used.
Disadvantage: Similar to the uniform area method.
- Local Mean and Local Standard Deviation (LMLSD)
Main idea: Assume that the image is composed of a large number of uniform small blocks, and the noise is mainly additive noise. Using the concepts of local mean and local standard deviation, the image is divided into many small blocks, and then the standard deviation of these sub-blocks is calculated as the size of the local noise, and the average value of the local standard deviations containing the most sub-block interval is selected as the entire image. Best noise estimate.
Disadvantages: Only valid for white Gaussian noise. For images with random Gaussian noise, the signal is disturbed by noise.
- Spatial / spectral dimension decorrelation (SSDC)
The main idea: It is a noise assessment method specifically for hyperspectral images. It uses the characteristics of high correlation between the spatial and spectral dimensions of hyperspectral images, removes highly correlated signals through multivariate linear regression, and uses the resulting residual image pairs. Noise is estimated.
Evaluation: This method is less affected by the type of ground cover and can be automatically executed. It is a relatively stable method for evaluating hyperspectral image noise.
3. Common methods of dimensionality reduction of hyperspectral image data
The large number of spectral bands in hyperspectral remote sensing images provide extremely rich information for feature extraction, which is conducive to finer feature classification. However, the increase of bands will also lead to information redundancy and increased data processing complexity. .Data dimensionality reduction meets the following conditions: retain the characteristic information of the data as much as possible; remove data redundancy and correlation.
Dimension reduction is mainly performed from two aspects of feature selection and feature extraction .
3.1 Feature selection
Feature selection is spectral combination, that is , selecting a band from the original spectral band number, the number of possible spectral combinations is NM( N> M)
Here you can use the Band Index method to reduce the spectral band selection.
Band Index method: * Hyperspectral remote sensing images are divided into K groups (such as shortwave light, visible light, near-infrared) according to the correlation.
0 comments:
Post a Comment