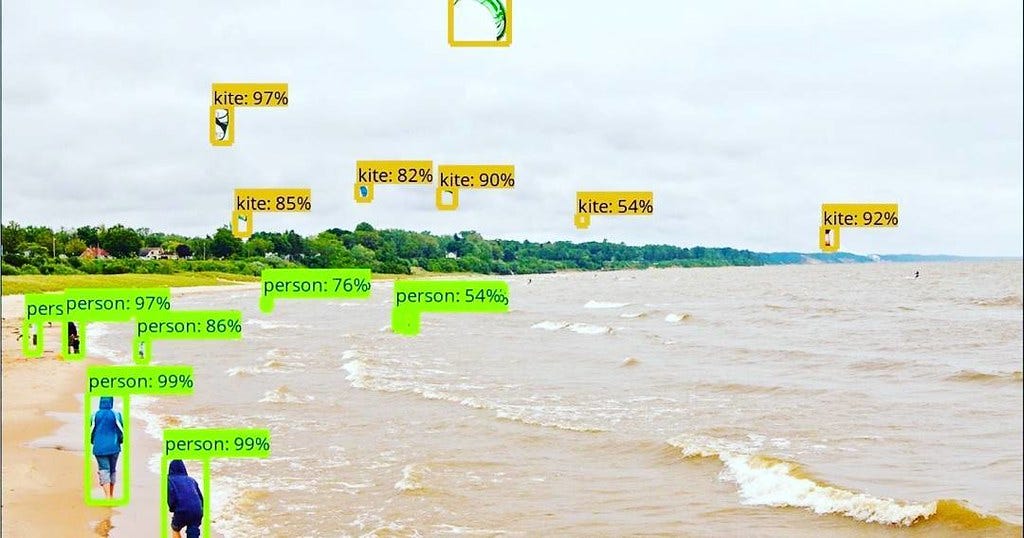
Just a few days ago Google AI launched an object detection competition on Kaggle called the Open Images Challenge. It’s great to see since the computer vision community hasn’t had such a new massive competition in a while.
For several years, ImageNet was the “gold standard” competition in computer vision. Many teams competed every year to get the lowest error rate on the ImageNet dataset. Thanks to deep learning, we’ve recently seen massive advances in the task of image recognition, even surpassing human level accuracy. In the graph below we can really see how having such a competition as large as ImageNet helps accelerate the research in the field, especially in the first few breakthrough years, beginning in 2012.
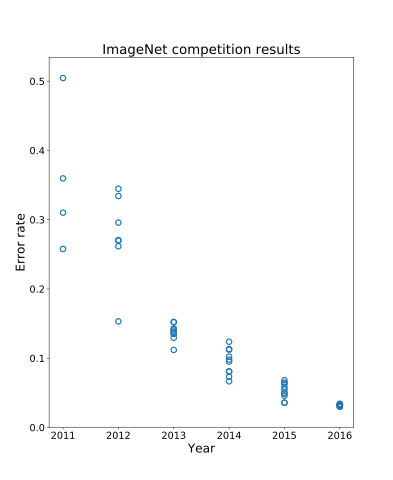
ImageNet was a huge competition, with 1000 different classes and 1.2 Million training images! The sheer scale of the data is really what made ImageNet so challenging. A very important thing that we have gotten out of doing such large scale competitions (in addition of course to learning how to classify images very well) is the feature extractors that we can use for other tasks. Feature extraction networks pre-trained on ImageNet are used in many other computer vision tasks including object detection, segmentation, and tracking. In addition, the general style or design of the network is often adopted for these other tasks. For example, shortcut connections were originally used in the 2015 winning ImageNet entry, and have since been used in the vast majority of CNNs in computer vision! This is a great thing, when we can work on one, simpler task and it has huge carryovers to more complex but related tasks.
Google AI’s new object detection competition, hosted on Kaggle, is a step in that positive direction. Thus far, the COCO detection challenge has been the big one for object detection. But, in comparison to ImageNet, it’s quite small. COCO only has 80 categories and 330K images. It’s not nearly as complex as what you would see in the real world. Many practitioners often find object detection in the wild to be extremely challenging. At least ImageNet had a large enough dataset and enough classes that it became very useful for pre-training, and using the networks for transfer learning. Perhaps with a large enough dataset our object detectors can become just as great for transfer learning.
That’s where this new competition comes in! Google AI has publicly released the Open Images dataset. Open Images follows the tradition of PASCAL VOC, ImageNet and COCO, now at an unprecedented scale.
The Open Images Challenge is based on the Open Images dataset. The training set of the Challenge contains:
- 12M bounding-box annotations for 500 object classes on 1.7M training images
- Images of complex scenes with several objects–an average of 7 boxes per image
- Highly varied images that contain brand new objects like “fedora” and “snowman”
- Class hierarchy that reflects the relationships between classes of Open Images
In addition to the Object Detection track, the Competition also includes a Visual Relationship Detection track to detect pairs of objects in particular relations, e.g. “woman playing guitar,” “beer on table,” “dog inside car”, “man holding coffee”, etc. You can find out more about the dataset here. The awesome thing about this dataset that you can really see right away from that link is the variety. There’s a nice visualization of all 600 classes in the dataset here where you can see the breakdown and hierarchy of the classes; it’s really quite diverse. We can also observe that there’s a very wide range of class frequencies. This means that we can’t just naively treat all of the classes equally; we are forced us to take into account the class distributions, much more real-world style! The robustness of this dataset definitely brings us closer to creating models more useful for deployment in the wild.
If you like prizes, there’s also a $30,000 prize pool! Plus, the results of the Challenge will be presented at a workshop at the European Conference on Computer Vision 2018. It’s being held in Munich, Germany which will surely be a nice trip!
It’s also great to see the competition being hosted on Kaggle. The Kernels of the challenge often end up being a huge source of knowledge from seeing the competitors many different approaches. Such a large scale and complex challenge will hopefully bring out the best research and new ideas that can be applied across the field of Computer Vision, just like ImageNet did!
কিছু দিন আগে গুগল এআই ওপেন ইমেজস চ্যালেঞ্জ নামে একটি কাগজতে একটি বিষয় সনাক্তকরণ প্রতিযোগিতা শুরু করে। কম্পিউটার দর্শন সম্প্রদায়ের কিছুক্ষণের মধ্যে এত নতুন বিশাল প্রতিযোগিতা হয়নি বলে এটি দেখতে দুর্দান্ত।
বেশ কয়েক বছর ধরে, চিত্রকল্প কম্পিউটার দৃষ্টিভঙ্গিতে "স্বর্ণের মান" প্রতিযোগিতা ছিল। ইমেজনেট ডেটাসেটের সর্বনিম্ন ত্রুটি হার পেতে অনেক দল প্রতি বছর প্রতিযোগিতা করেছিল। গভীর জ্ঞানার্জনের জন্য ধন্যবাদ, আমরা সম্প্রতি চিত্রের স্বীকৃতি দেওয়ার কাজটি এমনকি মানব স্তরের যথার্থতাকে ছাড়িয়ে গিয়ে ব্যাপক অগ্রগতি দেখেছি। নীচের গ্রাফের মধ্যে আমরা সত্যিই দেখতে পারি যে ইমেজনেটের মতো এত বড় একটি প্রতিযোগিতা কীভাবে ক্ষেত্রের গবেষণাকে বিশেষ করে প্রথম কয়েক যুগান্তকারী বছরগুলিতে, যা ২০১২ সাল থেকে শুরু করে তত্পরতা ত্বরান্বিত করতে সহায়তা করে।
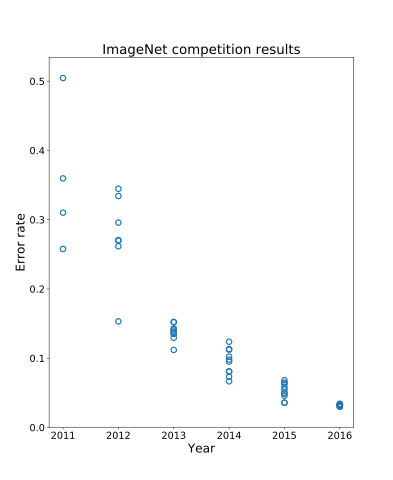
ইমেজনেট ছিল এক বিশাল প্রতিযোগিতা, যার সাথে 1000 বিভিন্ন ক্লাস এবং 1.2 মিলিয়ন প্রশিক্ষণের চিত্র রয়েছে! ডেটার নিখুঁত স্কেল যা সত্যই ইমেজনেটকে এত চ্যালেঞ্জযুক্ত করে তুলেছিল। একটি খুব গুরুত্বপূর্ণ বিষয় যা আমরা এত বড় আকারের প্রতিযোগিতা করতে পেরেছি (চিত্রগুলি খুব ভালভাবে শ্রেণিবদ্ধ করতে শেখার পাশাপাশি) বৈশিষ্ট্য নিষ্কর্ষক যা আমরা অন্যান্য কাজের জন্য ব্যবহার করতে পারি। ইমেজনেটে প্রাক-প্রশিক্ষিত বৈশিষ্ট্য নিষ্কাশন নেটওয়ার্কগুলি অবজেক্ট সনাক্তকরণ, বিভাগকরণ এবং ট্র্যাকিং সহ অন্যান্য অনেক কম্পিউটার দৃষ্টি কার্যগুলিতে ব্যবহৃত হয়। এছাড়াও, অন্যান্য অন্যান্য কাজের জন্য প্রায়শই নেটওয়ার্কের সাধারণ স্টাইল বা নকশা গ্রহণ করা হয়। উদাহরণস্বরূপ, শর্টকাট সংযোগগুলি মূলত ২০১৫ সালের বিজয়ী চিত্রনাট এন্ট্রিতে ব্যবহৃত হয়েছিল এবং এরপরে কম্পিউটার ভিউজে প্রচুর পরিমাণে সিএনএন ব্যবহার করা হয়েছে! এটি একটি দুর্দান্ত জিনিস, যখন আমরা একটির উপর কাজ করতে পারি,
গুগল এআই-এর নতুন অবজেক্ট সনাক্তকরণ প্রতিযোগিতা, কাগলকে হোস্ট করা, সেই ইতিবাচক দিকের এক ধাপ। এখনও অবধি, বস্তু সনাক্তকরণের জন্য কোকো সনাক্তকরণ চ্যালেঞ্জই সবচেয়ে বড়। তবে, ইমেজনেটের তুলনায় এটি বেশ ছোট। COCO- এ কেবল 80 টি বিভাগ এবং 330 কে চিত্র রয়েছে। আপনি বাস্তব বিশ্বে যা দেখবেন এটি এতটা জটিল নয়। অনেক অনুশীলনকারী প্রায়শই বন্যের মধ্যে বস্তু সনাক্তকরণ অত্যন্ত চ্যালেঞ্জিং বলে মনে করেন। কমপক্ষে ইমেজনেটে একটি বৃহত পরিমাণে ডেটাসেট এবং পর্যাপ্ত ক্লাস ছিল যা এটি প্রাক-প্রশিক্ষণের জন্য, এবং স্থানান্তর শিক্ষার জন্য নেটওয়ার্কগুলি ব্যবহারের জন্য খুব দরকারী হয়ে ওঠে। সম্ভবত একটি বৃহত পরিমাণে ডেটাসেটের সাহায্যে আমাদের অবজেক্ট ডিটেক্টরগুলি স্থানান্তর শেখার জন্য ঠিক তত দুর্দান্ত হয়ে উঠতে পারে।
এই নতুন প্রতিযোগিতা আসে যেখানে! গুগল এআই প্রকাশ্যে ওপেন ইমেজগুলি ডেটাসেট প্রকাশ করেছে । উন্মুক্ত চিত্রগুলি এখন অভূতপূর্ব স্কেলে পাস্কাল ভিওসি, ইমেজনেট এবং সিওসিওর followsতিহ্য অনুসরণ করে।
ওপেন ইমেজস চ্যালেঞ্জটি ওপেন চিত্রগুলি ডেটাসেটের উপর ভিত্তি করে। চ্যালেঞ্জের প্রশিক্ষণ সংস্থায় রয়েছে:
- 1.7M প্রশিক্ষণ চিত্রগুলিতে 500 অবজেক্ট ক্লাসের জন্য 12 এম বাউন্ডিং-বাক্স টিকা
- বেশ কয়েকটি অবজেক্ট সহ জটিল দৃশ্যের চিত্র image প্রতি চিত্রে গড়ে 7 টি বাক্স
- অত্যন্ত বৈচিত্রময় চিত্রগুলিতে ব্র্যান্ডের নতুন অবজেক্ট যেমন "ফেডোরা" এবং "স্নোম্যান" রয়েছে
- ক্লাস শ্রেণিবিন্যাস যা ওপেন চিত্রগুলির ক্লাসগুলির মধ্যে সম্পর্ককে প্রতিফলিত করে
অবজেক্ট ডিটেকশন ট্র্যাক ছাড়াও, প্রতিযোগিতায় একটি বিশেষ সম্পর্কগুলির মধ্যে জোড়া জোড়া সনাক্ত করার জন্য একটি ভিজ্যুয়াল রিলেশনশিপ সনাক্তকরণ ট্র্যাকও অন্তর্ভুক্ত থাকে, যেমন "মহিলা গিটার বাজানো," "টেবিলের উপর বিয়ার," "গাড়ীর ভিতরে কুকুর", "কফি রাখার মানুষ" , ইত্যাদি আপনি এখানে ডেটাসেট সম্পর্কে আরও জানতে পারেন । এই ডেটাসেট সম্পর্কে দুর্দান্ত জিনিসটি যা আপনি সত্যিই সেই লিঙ্কটি থেকে সরাসরি দেখতে পেতে পারেন তা হ'ল বৈচিত্র্য। সেখানে ডেটাসেটে সব 600 ক্লাস একটা চমৎকার কল্পনা এর এখানে যেখানে আপনি ভাঙ্গন এবং ক্লাস অনুক্রমের দেখতে পারেন; এটি সত্যিই বেশ বৈচিত্র্যময়। আমরা পর্যবেক্ষণ করতে পারেনযে ক্লাস ফ্রিকোয়েন্সি একটি খুব বিস্তৃত আছে। এর অর্থ হ'ল আমরা কেবল নির্লজ্জভাবে সমস্ত শ্রেণীর সাথে সমানভাবে আচরণ করতে পারি না; আমরা আমাদের ক্লাস বিতরণগুলি আমলে নিতে বাধ্য করি, আরও অনেক বেশি বাস্তব-বিশ্বের স্টাইল! এই ডেটাসেটের দৃust়তা অবশ্যই বন্যগুলিতে স্থাপনার জন্য আরও দরকারী মডেল তৈরি করতে আমাদের আরও কাছে এনেছে।
আপনি যদি পুরষ্কার পছন্দ করেন তবে একটি $ 30,000 পুরষ্কার পুলও রয়েছে! এছাড়াও, চ্যালেঞ্জের ফলাফল কম্পিউটার ভিশন 2018 এর ইউরোপীয় সম্মেলনে একটি কর্মশালায় উপস্থাপিত হবে । এটি জার্মানির মিউনিখে অনুষ্ঠিত হচ্ছে যা অবশ্যই একটি দুর্দান্ত ভ্রমণ হবে!
কেগল-তে প্রতিযোগিতাটি অনুষ্ঠিত হচ্ছে তা দেখেও দুর্দান্ত। প্রতিদ্বন্দ্বীদের বিভিন্ন রকমের দৃষ্টিভঙ্গি দেখে জ্ঞানের এক বিশাল উত্স হিসাবে চ্যালেঞ্জটির কার্নেলগুলি প্রায়শই শেষ হয়। এত বড় একটি স্কেল এবং জটিল চ্যালেঞ্জ আশাবাদী যে কম্পিউটার উইশন এর ক্ষেত্র জুড়ে প্রয়োগ করা যেতে পারে এমন সেরা গবেষণা এবং নতুন ধারণা নিয়ে আসবে, যেমন ইমেজনেট করেছিল!
0 comments:
Post a Comment